2021 Year in Review
Quantifying Uncertainty in Mission-Critical AI Systems
The Department of Defense (DoD) and the intelligence community are adopting more artificial intelligence (AI) technology. However, many machine learning (ML) models within AI applications cannot accurately estimate or communicate the certainty of their inferences about real-world data. Downstream AI components and human users may make decisions about inferences they cannot know are bad.
The SEI is leveraging experience in human-computer interaction, enterprise-level infrastructure, and AI to develop new techniques and tools to quantify, identify, and rectify uncertainty in ML models. Improving ML uncertainty estimation supports the robust-and-secure pillar of AI engineering, a field spearheaded by the SEI.
We intend our methods, metrics, visualizations, and algorithms to be matured and eventually equip practitioners with tools to build more robust ML models.
Senior Machine Learning Researcher, SEI AI Division
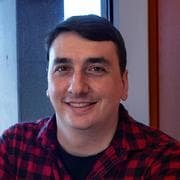
Quantifying uncertainty is first. According to SEI senior research scientist Eric Heim, deep neural network models tend toward overconfidence and require calibration, but current calibration methods are error prone and often yield poor confidence estimations.
In 2021, Heim and his colleagues developed metrics to evaluate the calibration of ML models against mission context. Better calibrated ML models can supply more accurate, context-sensitive estimates of confidence. This ability could help an intelligence operator, for example, decide when to trust an ML system’s identification of a vehicle from satellite photos. Heim and his colleagues will release the calibration metrics, and the evaluation code that produced them, on the SEI’s GitHub site.
Calibration evaluation is the first step toward detecting ML model uncertainty, determining its cause, and mitigating it. To achieve these challenging goals, Heim’s team works with Carnegie Mellon University ML experts Aarti Singh and Zachary Lipton. “We intend our methods, metrics, visualizations, and algorithms to be matured and eventually equip practitioners with tools to build more robust ML models,” said Heim. Such tools could make mission-critical AI systems more reliable and transparent, safer, and faster to update and deploy in DoD and intelligence operational environments.
Researchers
Eric Heim (project lead), Jay Palat, Carol Smith, Jon Helland, John Kirchenbauer, Jacob Oaks
More on Artificial Intelligence Engineering from the 2021 Year in Review

Operationalizing Responsible Artificial Intelligence
The Defense Innovation Unit collaborated with the SEI to operationalize ethical AI principles.
READ MORE
New Artificial Intelligence Division to Advance the Discipline
The AI Division researches the practical design and implementation of AI.
READ MORE
AI Engineering: Building the Discipline and Growing an Ecosystem
The SEI has begun formalizing the field of AI engineering, much as it did for software engineering in the 1980s.
READ MORE