2022 Year in Review
Applying Causal Learning to Reduce Testing Times and Costs
The Department of Defense (DoD) is focused on deploying the latest technologies more rapidly to make next-generation capabilities available to personnel in the field as quickly as possible. Many of these technologies require rigorous testing and evaluation involving live experiments in physical environments, which are often too costly and time consuming to sustain rapid development and deployment. One solution is to test with simulators, an environment whose realism the DoD is constantly seeking to improve.
The SEI is collaborating with the DoD's Director of Operational Test and Evaluation (DOT&E) and with the Naval Undersea Warfare Center (NUWC) on a novel approach to improve simulations for testing advanced systems. The SEI team is taking data from NUWC’s in-water testing and comparing it to output from simulators using various methods, including multivariate outlier analysis. The technique identifies gaps—outliers in the combined data set—between simulations and real-world data.
“The novelty of our approach is to integrate multivariate outlier analysis with modern causal learning to create richer data for analysis,” explained Bob Stoddard, the SEI’s project lead. Causal learning identifies direct causes of a particular outcome to make sense of a large data set, in this case the interconnections between a weapon system, its environment, events that occur during testing, and the ultimate outcomes. A preliminary demonstration of causal learning on an NUWC in-water testing plan eliminated noncausal experimental factors, roughly doubling the test's efficiency.
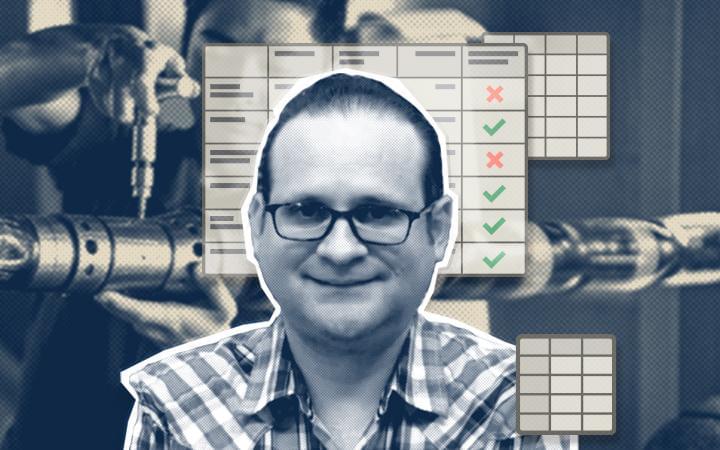
The SEI’s R&D into applying causal learning … represents a strong step toward achieving our goal of engendering a lifecycle approach to the verification and validation of modeling and simulation.
Dr. Jeremy S. WernerChief Scientist, DOT&E, Department of Defense
Disciplines including medical research, economics, and social science already use causal learning. The SEI’s work with DOT&E and NUWC is innovating the combination of this approach with outlier analysis to improve engineering testing and evaluation. This groundbreaking work is in its early stages, but it aims to give NUWC deeper insights into its in-water testing data to make more effective use of simulators, saving money and development time.
“The SEI’s R&D into applying causal learning to discover differences between live test and simulation in support of model refinement represents a strong step toward achieving our goal of engendering a lifecycle approach to the verification and validation of modeling and simulation,” said Dr. Jeremy S. Werner, DOT&E chief scientist. “That includes a live test, refine, predict feedback loop to bring live data and simulation into increasing harmony over time.”
RESEARCHERS
Bob Stoddard (project lead), Nanette Brown, Mike Konrad, Melissa Ludwick, David Shepard, Nicholas Testa